I Know What Sifflet Did Last Summer: Product Update

This summer, like every other season, our engineering teams have been hard at work, delivering high-value features designed with you in mind. By focusing on Sifflet’s three core pillars, our latest upgrades will further empower you to catalog, monitor, and trace all your data assets seamlessly in one place—Sifflet.
I) Accessibility:
At Sifflet, we believe data should be reliable and accessible for everyone, without barriers. That's why we're bringing data observability insights directly to where you work with your data. Our Chrome extension was just the beginning, giving BI users a smooth way to access these insights.
Data Catalogs
We're excited to take the next step by integrating with Data Catalogs, making it easier for you to access Sifflet’s quality metrics right where you need them. Whether through partnerships or native/API integrations, we're collaborating with Atlan, Alation, Castor, and Data Galaxy to bring this vision to life.

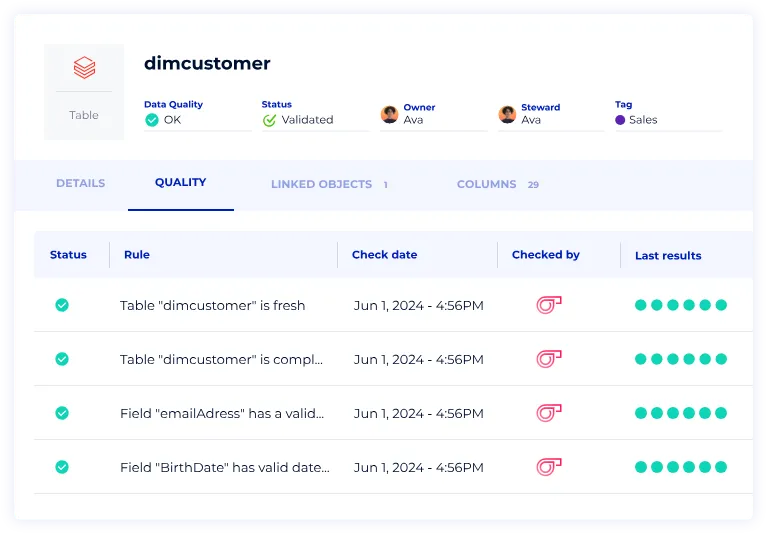
To make data observability accessible to as many data users as possible, providing business context and powerful discovery capabilities is essential. We started this journey with our Catalog, Domains, Tags, and Descriptions, and we're continuously enhancing these features.
Rich Context
We’re excited to introduce Rich Text Descriptions for assets and Sifflet Monitors, allowing you to include images and even import markdown descriptions from dbt models. This means that every Sifflet user, regardless of their technical background, can easily find healthy assets, identify critical ones to monitor, and better understand the true impact of data incidents.
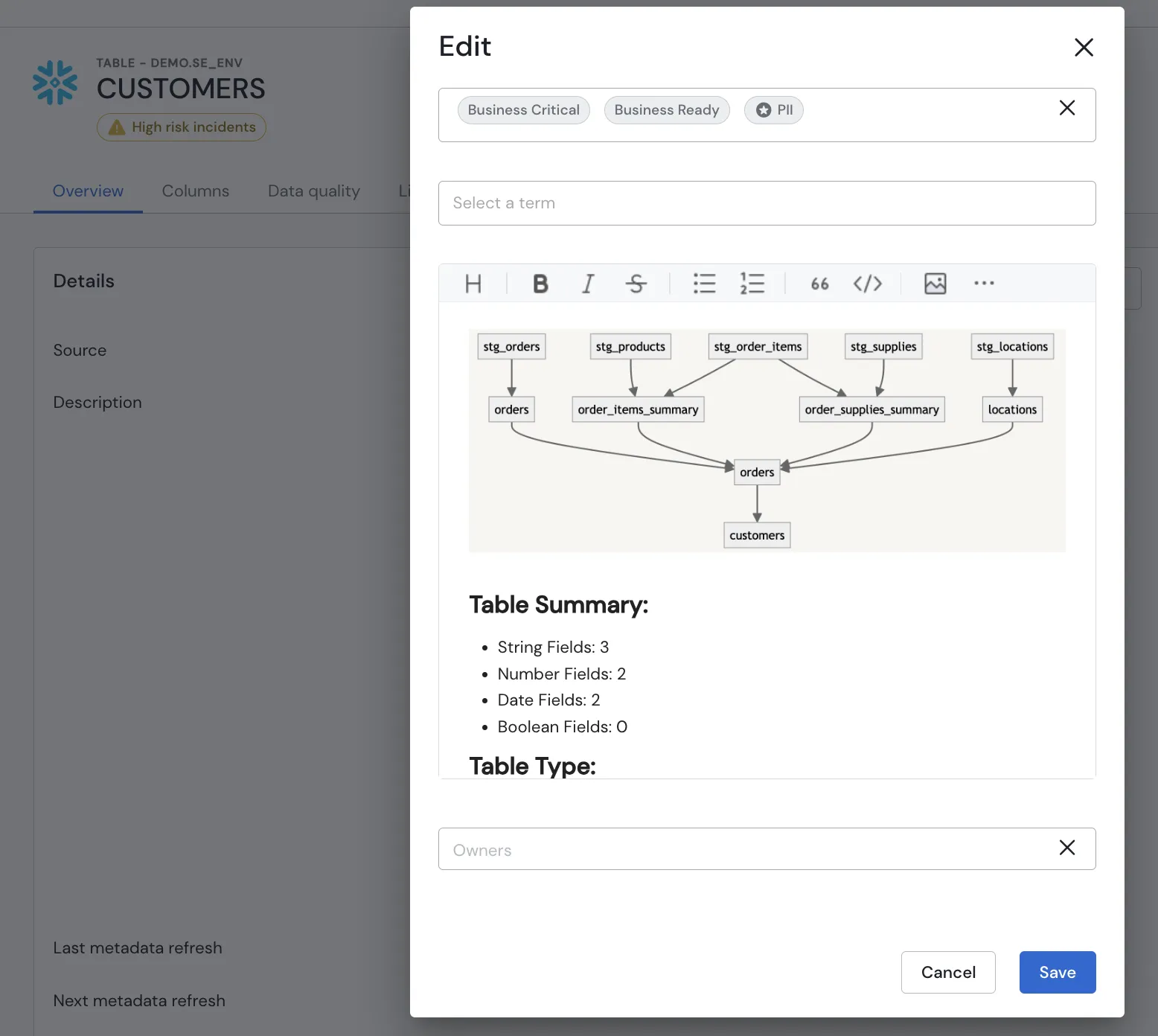
II) Reliability
Trust comes from the confidence that your data is being monitored effectively, allowing incidents to be flagged and resolved quickly. Here’s what we’ve been focusing on these past few months:
Comprehensive Handling
We’ve made significant improvements to ensure your data observability is as reliable as possible, empowering you to catch and address issues before they escalate.
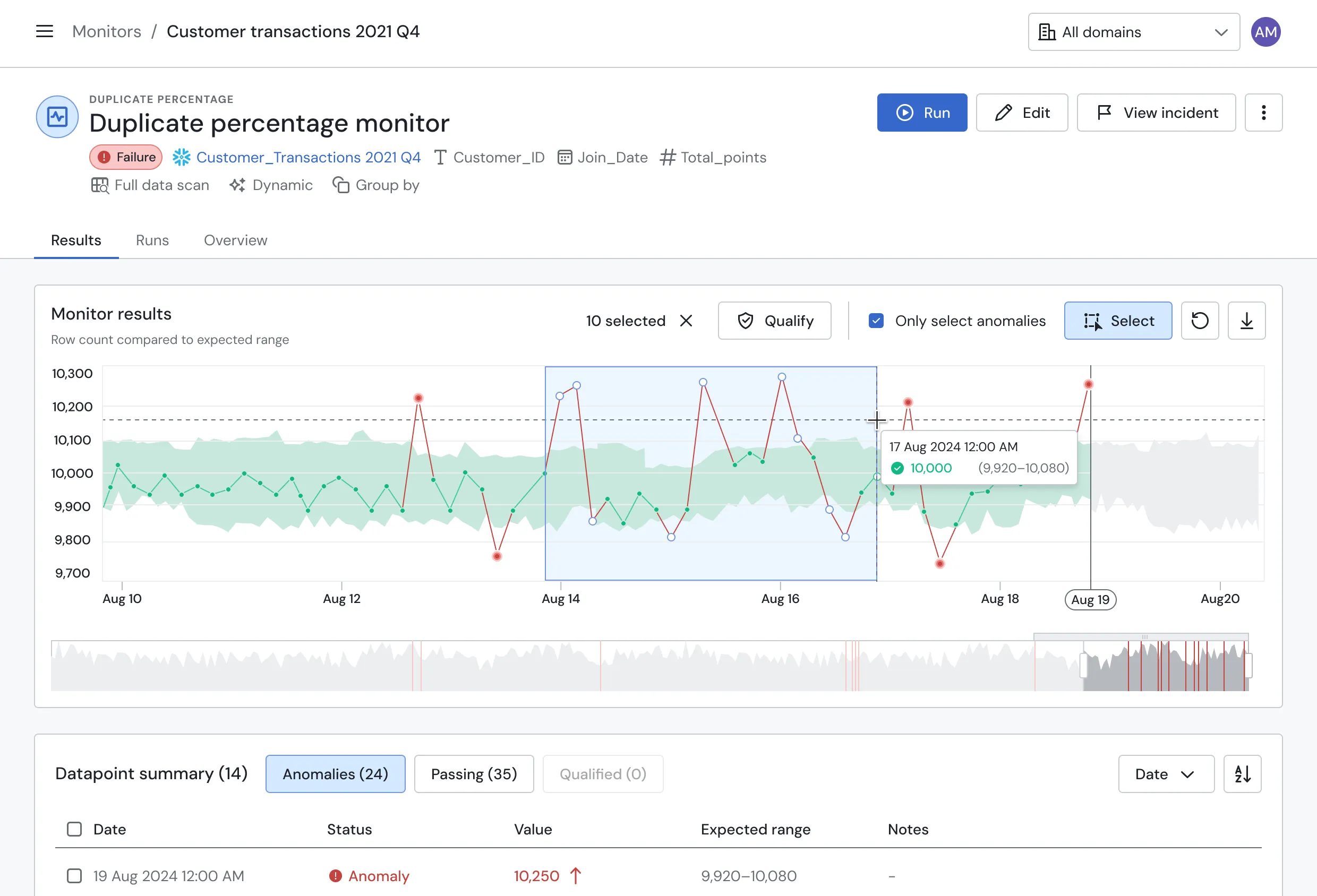
When an anomaly occurs, it’s rarely an isolated event. A single good data point doesn’t always mean previous issues have been resolved.
At Sifflet, we take pride in the accuracy of our models and qualifications, but we’re going a step further. We're now helping our customers scale their anomaly management by allowing them to batch qualify time ranges and compute monitor health based on all available qualifications.
Holidays and Special Business Dates
Many of our customers experience fluctuations in their data on specific days, like Christmas, stock market closure dates, or higher restaurant bookings on Valentine’s Day. To reduce noise and false positives, we’ve introduced a feature that allows you to exclude these dates from alerts by choosing from common calendars or specifying your own important business dates in advance.

Referential Integrity
At Sifflet, we’re continually expanding our monitoring capabilities to meet your evolving needs. One of our latest additions is the referential integrity monitor, designed to validate the logical dependencies between tables. For example, it ensures that every purchase in an order table has a valid customer ID, helping you maintain data accuracy and consistency.

Create Monitors with Code Even More Easily!
Many of our customers create hundreds, sometimes thousands, of monitors using code. To make this process smoother, we’ve enhanced our Data Quality as Code framework with new features, allowing you to deploy even more monitors with less effort and fewer lines of code. Now, with support for for-loops and templates, Sifflet customers can efficiently scale monitor deployment like never before.

III) Traceability
Data quality hinges on your ability to trace data, regardless of the data stack or product. That’s why we continue to enhance Sifflet’s holistic capabilities.
Synapse, Fivetran, MicroStrategy
Supporting a wide range of data solutions is a priority for us, as our customers work with diverse data stacks. Recently, we’ve expanded our support for BI, Data Warehouse, and Pipeline tools by integrating with MicroStrategy, Synapse (with both monitoring and lineage), and Fivetran—further shifting data observability left in the process.
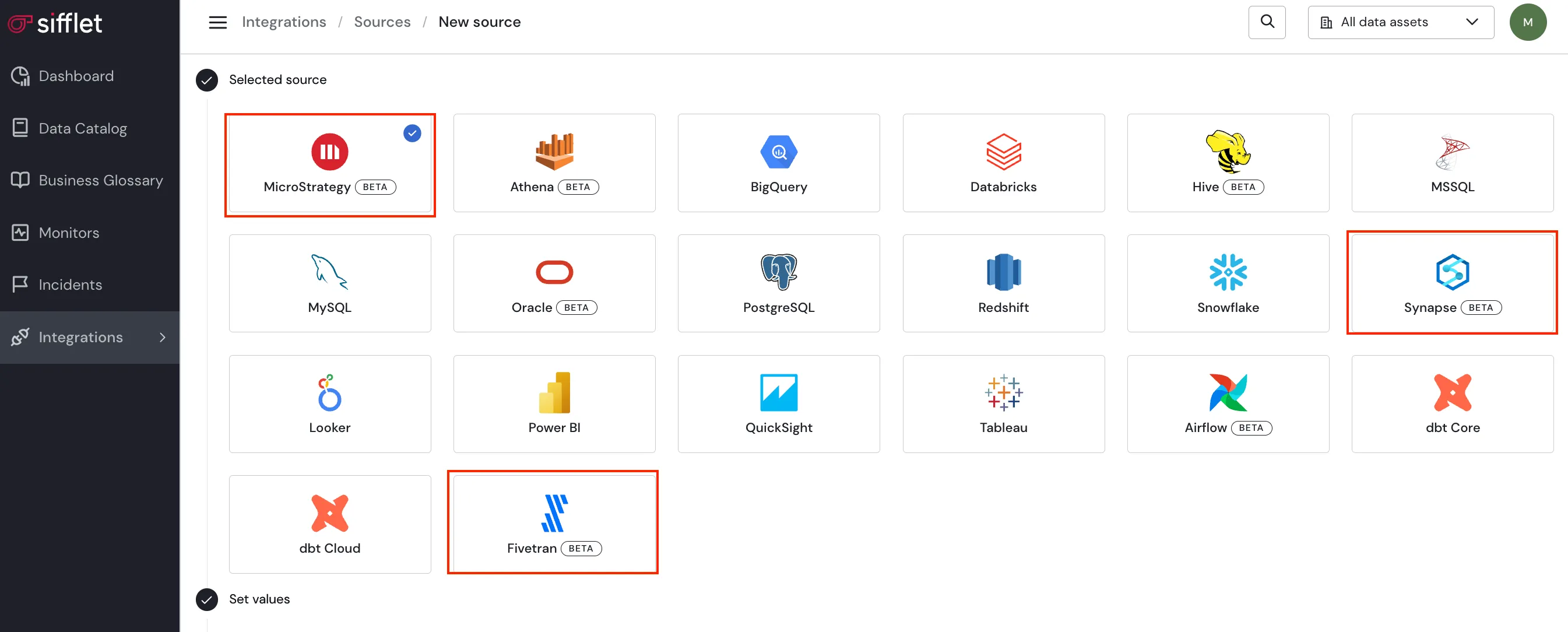
Everything in Sifflet: Universal Connector
While we prioritize native integrations at Sifflet, we recognize that it’s not always possible to connect with every tool. That’s where the Universal Connector comes in, enabling our customers to bring their own assets into Sifflet. This feature helps you gain deeper insights into the root causes and impacts of data incidents. Now, Sifflet customers can incorporate any source, pipeline, or data consumer—such as an AI model—into their Sifflet ecosystem!

Augmented Resolution
At Sifflet, we understand that data issues rarely exist in isolation; when something goes wrong, it often affects multiple areas. This summer, we’ve enhanced our incident support to empower our users to combine alerts and issues into a single collaborative ticket. This streamlined approach fosters teamwork and ensures a more effective resolution process!

Ticketing Systems
We understand that our customers have unique workflows and processes. Integrating incidents with your ticketing systems is crucial for ensuring data issues are addressed promptly and communicated effectively to the right stakeholders. This streamlined connection helps facilitate quick resolutions and keeps your team informed!
.webp)
Stay Tuned - More Coming Soon!
Data observability is an ever-evolving concept. As businesses expand their data use cases, the value of that data grows, making the demand for high-quality data more critical than ever. Data not only powers processes but can also be sold as a valuable asset. Recently, it has become essential for understanding that the increasing capabilities of AI and LLMs depend on quality data—poor models trained on bad data simply won’t deliver. The urgency to identify and resolve data incidents quickly has never been greater, and we’re committed to supporting you every step of the way!