Scale Your Data Observability: 3 Strategic Ways to Leverage Sifflet's Data Sharing
Go Beyond Monitoring: Integrate Observability Data for Stronger Governance, Custom Reporting, and Optimized Data Assets Management
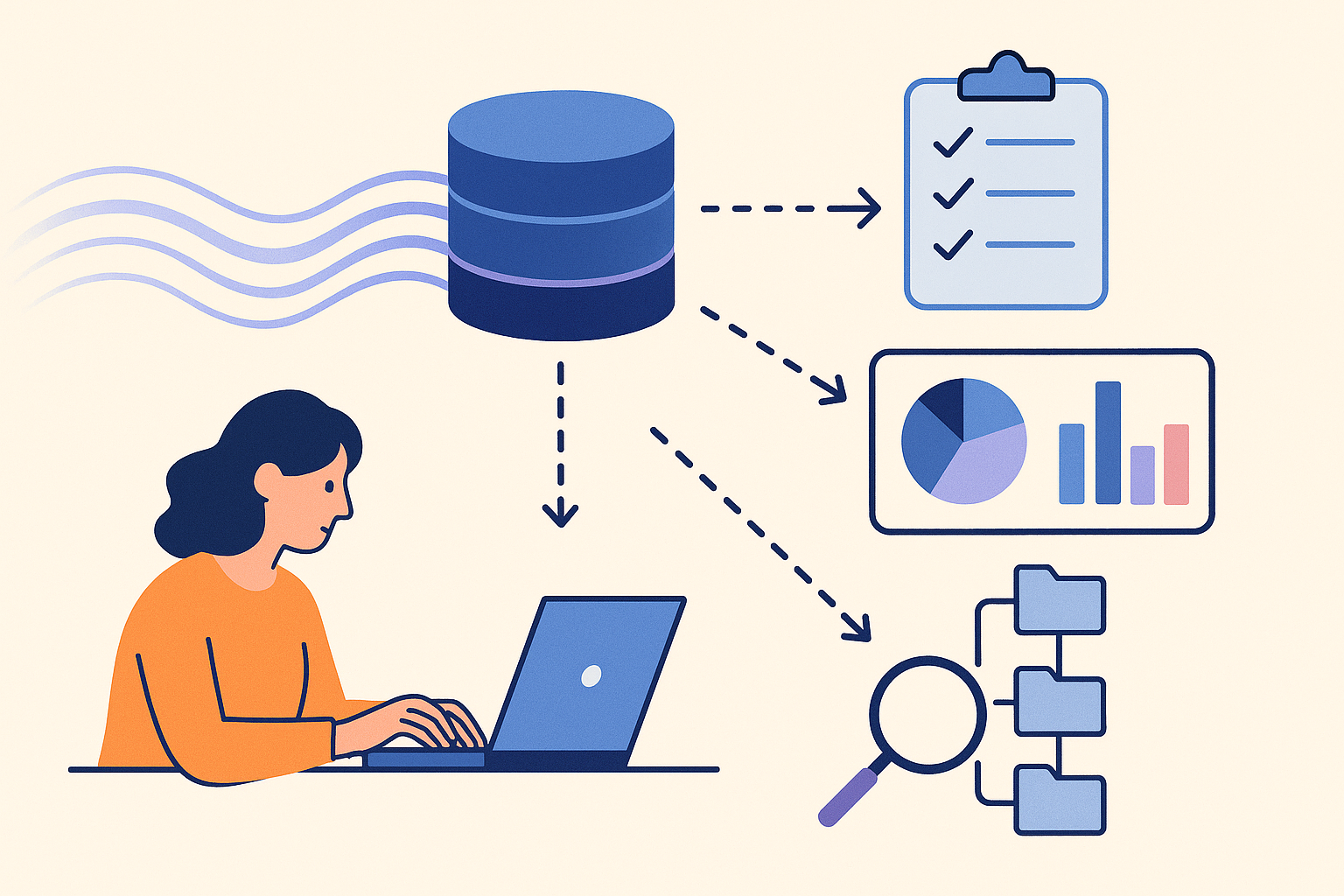
At Sifflet, our conversations with data teams consistently highlight a crucial challenge: achieving trustworthy data at scale isn't just about fixing isolated incidents. It requires building a sustainable data observability practice that integrates deeply within the organization. While Sifflet offers robust monitoring capabilities, true scalability comes from connecting observability data with your broader data ecosystem.
This is where Sifflet's Data Sharing feature plays a key role. It’s more than just an export; it’s an optimized pipeline delivering Sifflet's rich operational data and metadata (information about your data assets, monitors, lineage, tags, incidents, usage, etc.) directly into your own data platform – supporting Snowflake, BigQuery, and S3, with more destinations on the way. This data is refreshed every four hours, providing timely information for analysis, monitoring, and reporting.
Data Sharing transforms Sifflet from a standalone application into an integrated component of your data stack. This unlocks more effective ways to manage, govern, and understand your data landscape. It's a significant differentiator, enabling the integration and customization needed by data-mature organizations.
Let's explore three key ways Data Sharing helps you scale your data observability strategy.
1. Enforce Data Governance Policies and Standards Systematically
- The Challenge: Defining governance policies – like requiring owners and descriptions for critical data, or specific monitoring for staging tables – is the first step. Ensuring these policies are consistently applied across a large and dynamic data landscape is difficult to manage manually.
- How Data Sharing Helps: Sifflet gathers extensive metadata about your data assets and their configurations. By making this metadata available in your data warehouse via Data Sharing, you can build automated checks to monitor compliance with your specific governance rules.
- Why It Matters:
- Consistency: Ensure critical data assets consistently meet your defined standards.
- Risk Reduction: Quickly identify assets lacking proper documentation or ownership, which often pose higher risks.
- Scalability: Automate policy enforcement, moving beyond inefficient manual audits.
- Accountability: Gain clear visibility into governance adherence across different teams and domains.
- Practical Examples:
- Automatically detect tables tagged "business critical" that are missing an owner or a meaningful description.
- Verify that all tables within a specific schema (e.g.,
staging
) or matching a naming pattern (e.g.,stg_*
) have active freshness and volume monitors configured in Sifflet. - Ensure tables identified as containing PII are correctly tagged within Sifflet for proper handling.
- Leveraging the Data: A unique aspect here is the ability to use Sifflet itself to monitor the shared Sifflet data. You can create Sifflet monitors (using custom SQL or existing templates) that query the shared tables (
asset
,tag
,monitor
, etc. – see documentation for details) in your warehouse. When a monitor detects a policy violation, it triggers a standard Sifflet alert. This turns your governance framework into an active, automated monitoring system within your existing observability tool.
2. Build Custom Observability Reporting and KPIs
- The Challenge: Sifflet's built-in dashboards provide valuable operational views (and expect more enhancements soon!). However, different stakeholders often require tailored reports. Executives might need high-level health summaries by business unit, while platform teams may need detailed metrics on monitoring adoption or incident trends.
- How Data Sharing Helps: Data Sharing gives you direct access to granular data about monitors, incidents, assets, and their relationships. This allows you to use your company's preferred Business Intelligence (BI) tools (Tableau, Looker, Power BI, etc.) to build exactly the reports and dashboards you need.
- Why It Matters:
- Tailored Insights: Create reports customized for specific business units, data products, or strategic goals.
- Track ROI: Measure the effectiveness of your observability initiatives (e.g., are incidents in key areas decreasing? Is monitoring coverage improving?).
- Identify Hotspots: Pinpoint teams, domains, or pipelines experiencing frequent data issues or SLA breaches.
- Strategic Planning: Use concrete data to inform decisions about resource allocation for data quality improvements or infrastructure changes.
- Practical Examples:
- Visualize the percentage of critical tables monitored for key metrics (freshness, volume), broken down by data domain or owning team.
- Analyze incident trends (number, severity, resolution time) over time, filtered by data product or business impact.
- Report on the performance of critical data pipelines against defined SLAs.
- Develop custom data health scores based on monitoring results, incident frequency, and even governance compliance checks (from Pillar 1).
- Leveraging the Data: Connect your BI tool directly to the Sifflet data tables within your data platform. Use tables like
incident
,monitor_run
,monitor
,asset,
andtag
(refer to documentation for exact schemas) to create your analyses. With the data refreshed every four hours, your reports offer a timely and accurate view of your operational status.
3. Optimize Resources and Reduce Risk Through Usage Insights
- The Challenge: Data platforms naturally accumulate assets over time. Tables, pipelines, and dashboards created for past projects may no longer be in use, consuming resources and potentially increasing the attack surface or maintenance load. Identifying these safely is hard – deleting an unused asset that has hidden downstream dependencies can cause significant disruption. Conversely, assets assumed to be non-critical might become vital over time, creating risks if not adequately governed or monitored.
- How Data Sharing Helps: Sifflet maps the relationships between your data assets through lineage. By providing this lineage information alongside other metadata (like usage) via Data Sharing, you can analyze usage patterns and dependencies within your own data platform, helping you understand how assets are actually being used.
- Why It Matters:
- Cost Optimization: Confidently identify and review candidates for deprecation (unused tables, dashboards) to reduce storage, compute, and maintenance costs.
- Risk Mitigation: Address risks from unmanaged, unused assets, and uncover instances where non-production assets have become critical dependencies, ensuring they receive proper oversight.
- Improved Efficiency: Focus your team's efforts on maintaining and enhancing the data assets that provide the most value.
- Better Data Landscape Understanding: Gain a clearer, data-driven picture of asset utilization and interconnections to inform architectural decisions.
- Practical Examples:
- Identify "orphan" tables – those with no apparent downstream dependencies in Sifflet's lineage graph – making them primary candidates for deprecation review.
- Find tables that are part of critical data flows (based on lineage or usage) but lack essential monitoring coverage in Sifflet.
- Detect when a table tagged
staging
starts appearing as a source for assets taggedproduction
orcritical
, indicating a need to re-evaluate its status and controls. - Assess dashboard usage by analyzing their source table connections and monitoring status, helping identify potentially stale reports.
- Leveraging the Data: Analyze the
lineage
andasset_usage
tables to understand dependencies and usage patterns. Combine this with metadata from the different asset tables (such asasset
anddashboard
) and status information from themonitor
table. Querying this combined dataset within your platform allows you to accurately understand usage patterns based on connectivity and operational status.
Unlock the Strategic Value of Your Observability Data
Sifflet's Data Sharing feature helps you move beyond reactive monitoring towards a more proactive and strategic approach to data observability. By integrating Sifflet's operational and structural data into your environment, you gain significant flexibility and control.
We've covered three key ways this empowers your team:
- Automated Governance: Systematically enforce your data policies and standards.
- Custom Analytics: Build tailored reports and KPIs for deeper insights.
- Data Asset Optimization: Make informed decisions about your data assets based on usage insights enriched with lineage and metadata.
We provide this data through an optimized pipeline, refreshed every four hours, to leading platforms like Snowflake, BigQuery, and S3 (with more to come). Our philosophy is that Sifflet delivers maximum value as an open, integrated part of your data strategy. To that end, we are actively developing future enhancements for Data Sharing, including more aggregated views and optimized datasets, to make accessing and using this information even more straightforward.
This integrated approach allows you to actively manage, optimize, and govern your data ecosystem using rich, contextual insights.
Ready to put your observability data to work?
- Existing Sifflet Customers: Explore the documentation and the different tables available via Data Sharing and consider how the strategies discussed in this article can enhance your current operations. You can also reach out to your Customer Success Manager to discuss your specific goals and objectives related to governance, reporting, or optimization.
- Considering Sifflet? See how this deep integration capability enables a truly scalable, efficient, and data-driven observability practice. Reach out to our team to explore how Data Sharing can deliver strategic value for your organization.
Don't just monitor your data – use your observability data to drive smarter decisions, enforce stronger governance, optimize resource use, and build more trustworthy data products. Sifflet's Data Sharing is designed to help you achieve exactly that.