The Data Literacy Series - Data Quality
What is Data Quality? Why is it Important? How to measure it? and 5 best practices.
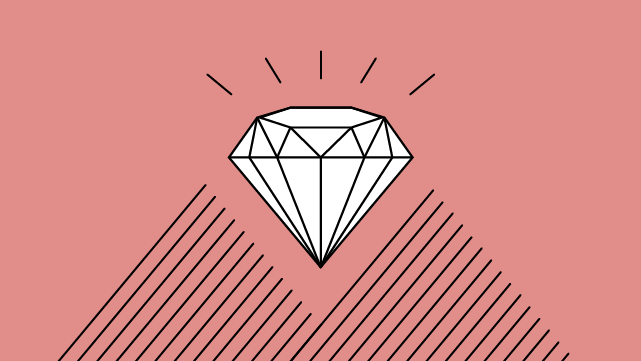
Today, everyone cares about data, and everyone is looking to invest in the latest tools to bring data-driven decision-making to their organizations. But before investing in some fancy data analytics tool, it’s vital to cover all the bases of the Modern Data Stack. This series aims to help everyone (yes, everyone!) get familiar with some of the most critical concepts in data.
We want to start this series with what we think is the most crucial challenge organizations face regarding data-driven decision-making: data quality. Teams are increasingly struggling to rely upon and trust their data, often making dashboards and insights generated from analytics void.
To explain how data quality can help teams regain trust in their data, we’ll answer the following questions:
- What is data quality?
- Why is data quality so important?
- How to measure data quality?
- How can you prevent bad data quality from undermining your business?
What is data quality?
Data quality - as the name suggests - refers to the health state of your data, and it is the most common method used to assess whether or not you can trust your data to drive business decisions. Data quality issues can occur long before the data starts being used - it can be impacted at any stage of the data pipeline, from ingestion to BI tools. And as data consumption increases within organizations, it becomes more and more crucial to trust the data to be reliable. Unreliable data can quickly become detrimental to the business. Think of missed opportunities, financial costs, customer dissatisfaction, failure to achieve regulatory compliance, inaccurate decision-making, etc.
Why is data quality so important?
Much like in the manufacturing or the service industries, lousy data quality means bad business. Let us give you an example. Brian is a data engineer at a grocery delivery company. During the first pandemic lockdown, business was thriving. But one day - despite all his best efforts to “manually” make sure that the data pipelines were error-free - the unforeseeable happened: data was accidentally duplicated. This error led to the duplication of order items, which in turn caused customers to receive double the quantity they ordered. On top of that, Brian only found out about this when customers started reporting the issue. It was already too late: the company locked in multiple thousands of dollars lost in this incident, which was a significant amount given the size of the business at the time.
Maybe you can relate to Brian (we know we can), or perhaps you have been getting by with the help of the many testing tools developed by your data engineering team to detect “recurrent” issues. We think the latter approach works fine, but it’s not scalable enough to tackle the challenges organizations face when dealing with data today.
But one thing we can all agree on is that if you can’t trust your data to make sound business decisions, you’ve got a problem.
How to measure data quality?
So how exactly do you know if your data isn’t reliable? There are a few metrics you can use to understand whether you can trust your data:
- Accuracy: Is the data correct, duplicate-free, and in the expected format?
- Completeness: Are there missing values, missing data records, or incomplete pipelines?
- Freshness / Timeliness: Is the data up-to-date?
- Relevance: Is the data intended for business use?
- Consistency: Is the data homogenous across the organization?
So, how can you prevent bad data quality from undermining your business?
Luckily, data quality can be assessed, tracked, and improved over time. Here are five best practices:
- Establish rigorous control of incoming data and introduce data profiling
- Have a clear definition of your business needs and KPIs to ensure that the data produced meets your business requirements.
- Evangelize data quality within your teams: data quality needs to be cultural and respected “religiously”. Define quality testing metrics and assign ownership over them for each of your projects.
- Invest in the right tools to monitor data quality at scale: as data consumption increases within organizations, short-term fixes won’t be enough to tackle data quality issues any longer.
- And finally, think of integrating data lineage and pipeline traceability to save time and effort when troubleshooting.
The future of data quality - Full Data Stack Observability
As the data journey from ingestion to consumption becomes increasingly complex, there are infinitely more opportunities for the data to break. On top of this, the amount of tools that teams use is growing, making it even more difficult to gain complete visibility into different parts of the data pipeline. These challenges are becoming issues within organizations, and data users struggle to trust their data.
Fortunately, a new approach to dealing with data quality has emerged in the past few years: data observability. Data observability can be defined as the ability of organizations to gain actionable insights regarding the health status of data. Data observability enables organizations to automatically monitor their data across critical ecosystem features, allowing data teams to identify and troubleshoot data quality issues and prevent them from breaking your analytics dashboard.
We believe that adopting Full Data Stack Observability is the best way to unlock the unlimited possibilities of data-driven decision-making without constantly worrying about the reliability of the data. Do you want to know more about Sifflet’s Full Data Stack Observability approach? Would you like to see it applied to your specific use case? Book a demo or get in touch for a two-week free trial!