How to Build a Modern Data Team
How to build the right data team for your company’s stage of data maturity.
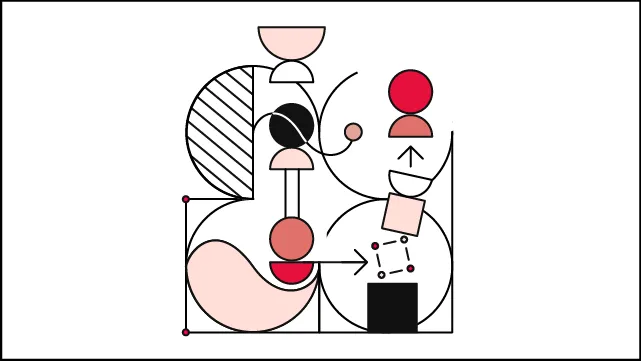
In a previous experience, I was asked to build a data team. The task was entirely new for me, so I did what anyone in my situation would do: I Googled it. I found many resources on the topic, but most of the blogs and articles mainly focused on the more technical aspects. Although some of the information was helpful, I still felt lost about where to begin.
This frustration is what motivated me to write this article. The aim here is to give pointers on what to focus on, how to prioritize, and other considerations that will benefit modern leaders of organizations taking their first steps towards data maturity.
I. Set the right objectives
The objectives of the data team should be set ahead of its implementation. They should involve the key stakeholders from different business divisions (engineering, product, marketing, finance, etc.), as many will ultimately be the consumers of the data products.
It is also crucial that the objectives of the data team reflect the company’s level of data literacy. Considering that you are building the data team from scratch, chances are your company is at the early stages of data maturity. More on this later.
The objectives of a data team can typically be categorized into the following :
- Exploratory: The company has some data, but you don’t know how to capitalize on it because you don’t know where to find it or whether you should trust it.
- Analytics: Your leadership is convinced that becoming data-driven is key to making better business decisions. There might already be some attempts to do analytics in Microsoft Excel or other tools, but you want to take data usage to the next level.
- Innovation: When you already have the necessary insights for making decisions, you think AI/ML will help you create your next differentiating edge; therefore, you want to start investing in that direction.
II. Define the organizational structure
Now that you’ve defined the business objectives, you should decide where the data team sits from a company organizational perspective. This step is crucial as it will put the proper foundation to avoid silos and unclear ownership. A few popular setups:
- Within Engineering: in some organizations like LinkedIn, the data team is part of engineering. Having seen a similar setup play out in the past, I think that Data and Engineering teams should work as partners and, therefore, with separate reporting lines. Creating a reporting dynamic between the two might jeopardize the efficiency of the collaboration and distance the Data team from the business.
- Within product: this makes sense when the product is tightly related to data and when the organization relies on data primarily for feature testing and other product analytics use cases.
- As an Independent Entity: reporting directly to the CEO or CFO. This makes sense for an organization that has: a. reached a good level of data maturity and company-wide data literacy, and b. a wide variety of well-defined use cases across different business functions, and is considering a “Data as a Product” type of approach catering to various business domains.
- Within a business entity: finance or marketing, e.g., This is usually the case for small data teams where the scope and objectives only pertain to this particular team (not recommended for larger companies).
III. Pick the appropriate Data Stack and Data Platform
With defined objectives and organizational reporting for your data team, you now need to consider several aspects: the company’s stage of data maturity, the data stack, and the data platform.
1.Stage of Data Maturity
- Basic: This is for any organization just getting started with data and looking to extract insights from data sources via an analytics tool. The size of the team can range from 1 (typically one of the founders or a Data Engineering hire) to a small 1–5 people data team.
- Intermediate: Data is utilized for various use cases: product, growth, business monitoring, etc. You already have an initial version of a Data Stack, and your Data team is growing.
- Advanced: Data is at the center of your company’s strategy and decision-making. Every department relies on data in their daily operations; use cases vary from Operational Analytics to advanced leveraging of AI and ML in product differentiation and goal setting.
2. The Data Stack
A Modern Data Stack (MDS) is a collection of tools and technologies that help businesses collect, transform, store and utilize data for analytics and ML use cases. The Modern Data Stack is cloud-based, modular and typically includes the following layers:
- Integration, ETL/ELT: where the data gets transported (and in some cases normalized and transformed) from source to storage. Think of tools like Fivetran, Stitch, and Airbyte.
- Storage: where the data is stored, typically a Cloud Data Warehouse or a Data Lake. Think of tools like Snowflake, Databricks, and BigQuery.
- Transformation & Modelling: where the raw data is transformed and put into the format, shape, and structure that makes it easily accessible for operational analytics. Think of tools like Dbt and Dataform.
- Business Intelligence/Data Visualization: where data is consumed, typically in a dashboard, chart, or table, and made accessible to business users. Think of tools like Tableau, Looker, and Mode.
- Workflow Orchestration: the “glue” that holds all these components together by allowing users to create, schedule, and monitor data pipelines.
- Reverse ETL: a reverse ETL is moving data from the data warehouse to other cloud-based business applications (CRM, Marketing, Finance tools, etc.) so it can be used for analytics purposes. Think of tools like Hightouch and Census.
- Data Observability: this is the “top of stack” layer that ensures the data is reliable and trustworthy across the whole stack. I am biased here as CEO and Cofounder of Sifflet.
3. The Data Platform
In general, data platforms can be:
- Centralized: This is arguably the most straightforward team structure to implement and a go-to for companies taking the first steps to become more data-driven. Both the team and the architecture are centralized here.
- Hybrid: The company is at a stage in its growth where multiple teams leverage data every day to make decisions. Data is treated as a product, and while the data team is centralized, efforts to further democratize the data within the organization have been made. There might be, in this case, the existence of “specialists,” often Data Analysts or Analytics Engineers within each business function that have enough technical skills to communicate and autonomously work with the data team while also having a business background.
- Fully Decentralized: The company is fully embracing decentralization in its Data platform, leveraging principles from concepts like the Data Mesh. The Data team (although, in this case, the idea of a traditional team is less relevant) mirrors the ubiquitous nature of the data. Each business domain can leverage the modular and self-serve-oriented nature of the data platform to unlock the most advanced data-powered cases. Think microservices but for a data platform, where domain expertise meets democratization of the data and its infrastructure.

IV. The hiring part
Let’s now focus on the Human Resources aspect. There are three core technical capabilities in a Data team: Data Engineering, Data Analytics, and Data Science. Other variations or combinations of these led to the emergence of roles like Analytics Engineer, ML Engineer, MLOps, BI Developer, etc. In the case of more data mature organizations, positions like DataOps, MLOps, DataSecOps, etc., are often sought after.
Let’s go through the three prominent roles in detail.
- Data Engineer: responsible for creating, scaling, and maintaining infrastructure that supports and produces the data. Skills to look for: Cloud technologies, Databases, ETL, Java, Python. More on this by Cord. co
Maxime Beauchemin wrote a series of great articles on Data Engineering as a career path, worth a read: The Rise of the Data Engineer and The Downfall of the Data Engineer. - Data Scientist: in charge of the creation, maintenance, and scaling of Data Science models using advanced statistics, data modeling, and ML techniques. Skills to look for: Statistical Analysis & Computing, Programming (Python, SQL, R), Machine Learning, Data Wrangling.
- Data Analyst: responsible for translating data into insights. The Data Analyst can link the end business need to the source data while also assessing the transformation and wrangling required. Skills to look for: SQL, business understanding (often understated but essential), Business Intelligence knowledge, creativity. Madison Schott, Analytics Engineer at WINC, published a series of articles on Analytics Engineering best practices that apply to Data Analytics.
Who should you hire first?
If you are just getting started, I advise you to follow the “less is more” rule. Start small by favoring “Full Data Stack” capabilities and keeping your data team’s objectives in mind; you can grow the team one member at a time as your necessities evolve.
At an early stage, the data team tends to focus on experimentation and initial POCs instead of bringing one big project into production. In this case, a Data Analyst or a Data Engineer with Analytics skills (Python, SQL, etc.) will be more valuable as a first hire. This person could work alongside Software Engineers on a first POC, which would help identify the first pipeline needs. This paves the way for the second hire, that should be someone with more Data & Architecture Engineering skills, to proceed with building the platform and making appropriate infrastructure choices. After this, further recruitment should be done according to ongoing projects.
What are the soft skills to look for?
Soft skills are essential when evaluating Data professionals. The Data practice is by default cross-functional; the Data team’s core mission is to help the business extract the maximum amount of value from data and become data-driven. Therefore, proximity to the business is indispensable. On the other hand, and especially in the early stages of Data Maturity, the data team also works closely with IT and Software engineering to ensure the robustness and sustainability of the Data Infrastructure. A good Data hire will have the following skills:
- Communication: the candidate needs to be a clear and efficient communicator with the ability to adapt to technical and non-technical audiences.
- Business knowledge: this is essential to ensure smooth adoption of data initiatives, help data consumers translate data into actionable insights, and know what to prioritize and when.
- Ethics and Security-first mindset: in the early stages, your processes are probably still vulnerable and lack data privacy and security. The right hire will ensure that best practices are researched and implemented to build the proper foundation for Data Protection and Compliance with Data regulations even at an early stage.
- Flexibility and Adaptability: the data team needs to be able to adapt to your company’s growth and the business considerations that might result from it, but also to the fast-paced nature of the data infrastructure and tooling industry.
V. Things to keep in mind
- Be business-focused: Most companies are not in the business of doing analytics for analytics, they grow through other means, and analytics is meant to serve growth.
- Do not underestimate internal evangelism — your organization needs strong executive buy-in and data leadership to foster data culture.
- Data leadership is essential to avoid a fractured data organization where business departments are not getting the help they need from the Data Team and are consequently constrained to hire other analysts.
- Communicate the expectations from the data team. Business unit leaders will get very excited about working with a data team. However — as resources will be limited at first — it is vital to set the right expectations from the beginning.
- Branding is key. Creating a coherent image of the data team will affect how the rest of the organization sees and interacts with it.
- Do not underestimate the technical debt. Learn about the past before the team existed. Many in-house debts (very long SQL queries, spreadsheets, etc.) were done as a temporary solution. It is essential to demonstrate the importance of changing existing data practices within the organization by showcasing valuable examples and case studies. This is especially important if trust needs to be restored within the team.
- Define clear success KPIs. As a general rule, these need to support ROI rather than directly impacting it.
Conclusion
Building a data practice is not only about making technological choices; and you will likely have to start with a first iteration and expect it to evolve as your business grows. Although there is no one size fits all approach, there are some best practices that I have gathered from my experience and the many conversations I have had with data leaders around the topic. Starting with the “why,” it is essential to set the right objectives for your modern data team by assessing your organization’s data maturity. Companies with different data maturity stages have different needs, which should drive the choices you make when creating your team. In addition, you need to define a clear role for the data team within the organization to avoid silos and unclear ownership. On top of this, you need to pick the best data stack and data platform for your organization. And while it might not be able to fit all considerations, this blog aims to provide you with an overview of best practices and a non-exhaustive list of recommendations to overcome some non-technical challenges that may arise when building a modern data team. While considering the non-technical challenges organizations face during the team creation phase, the technical aspects also deserve detailed attention. I will be discussing these at length in another blog.