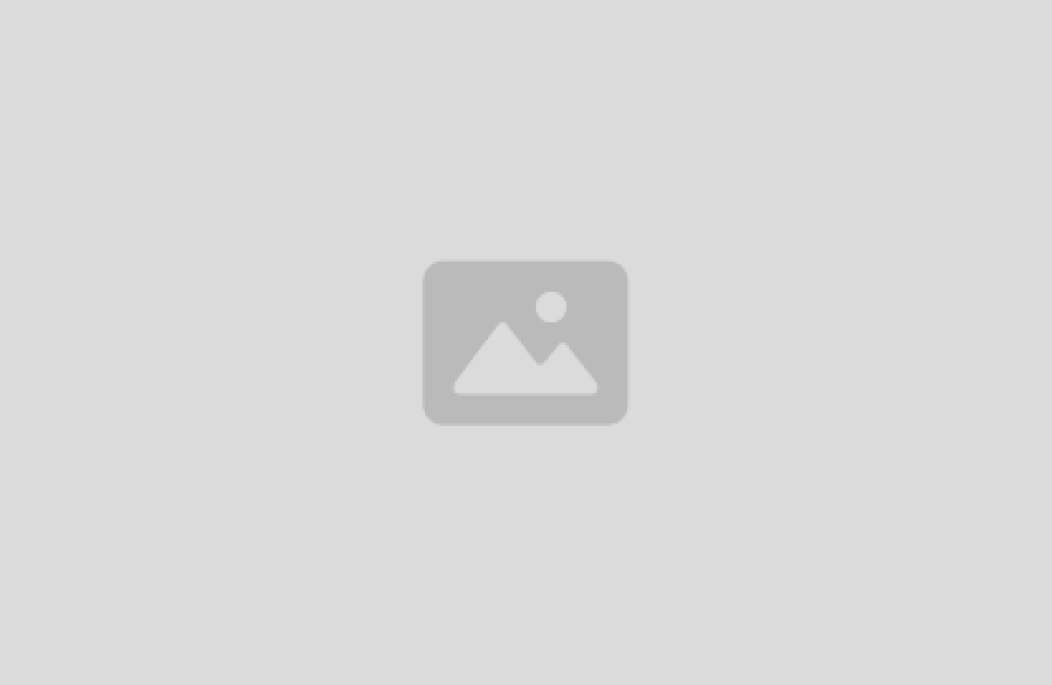
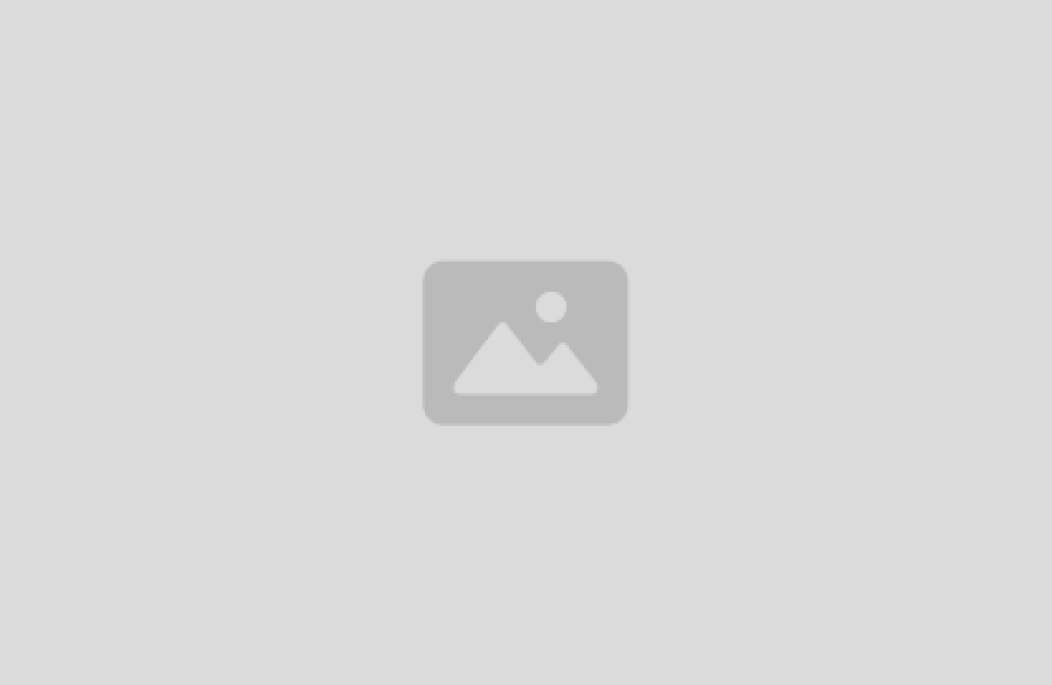
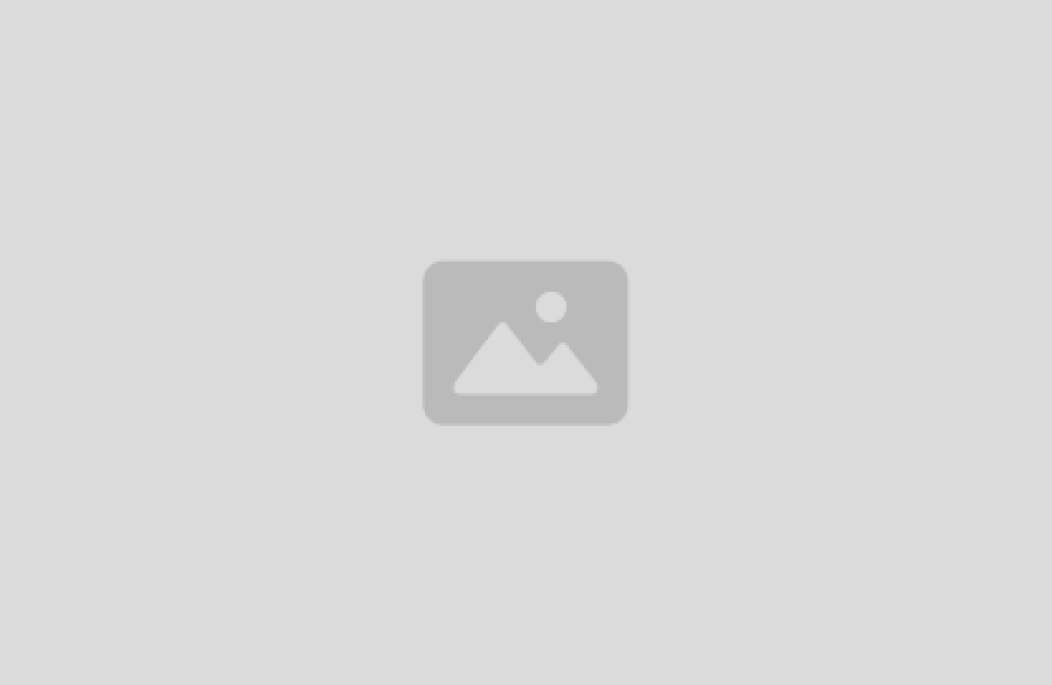
Discover more integrations
Get in touch CTA Section
Lorem ipsum dolor sit amet, consectetur adipiscing elit, sed do eiusmod tempor incididunt ut labore et dolore magna aliqua.
Frequently asked questions
Data-quality-as-code (DQaC) allows you to programmatically define and enforce data quality rules using code. This ensures consistency, scalability, and better integration with CI/CD pipelines. Read more here to find out how to leverage it within Sifflet
Yes, Sifflet leverages AI to enhance data observability with features like anomaly detection and predictive insights. This ensures your data systems remain resilient and can support advanced analytics and AI-driven initiatives. Have a look at how Sifflet is leveraging AI for better data observability here
AI enhances data observability with advanced anomaly detection, predictive analytics, and automated root cause analysis. This helps teams identify and resolve issues faster while reducing manual effort. Have a look at how Sifflet is leveraging AI for better data observability here
Data observability ensures data governance policies are adhered to by tracking data usage, quality, and lineage. It provides the transparency needed for accountability and compliance. Read more here.
Yes! While smaller organizations may have fewer data pipelines, ensuring data quality and reliability is equally important for making accurate decisions and scaling effectively. What really matters is the data stack maturity and volume of data. Take our test here to find out if you really need data observability.