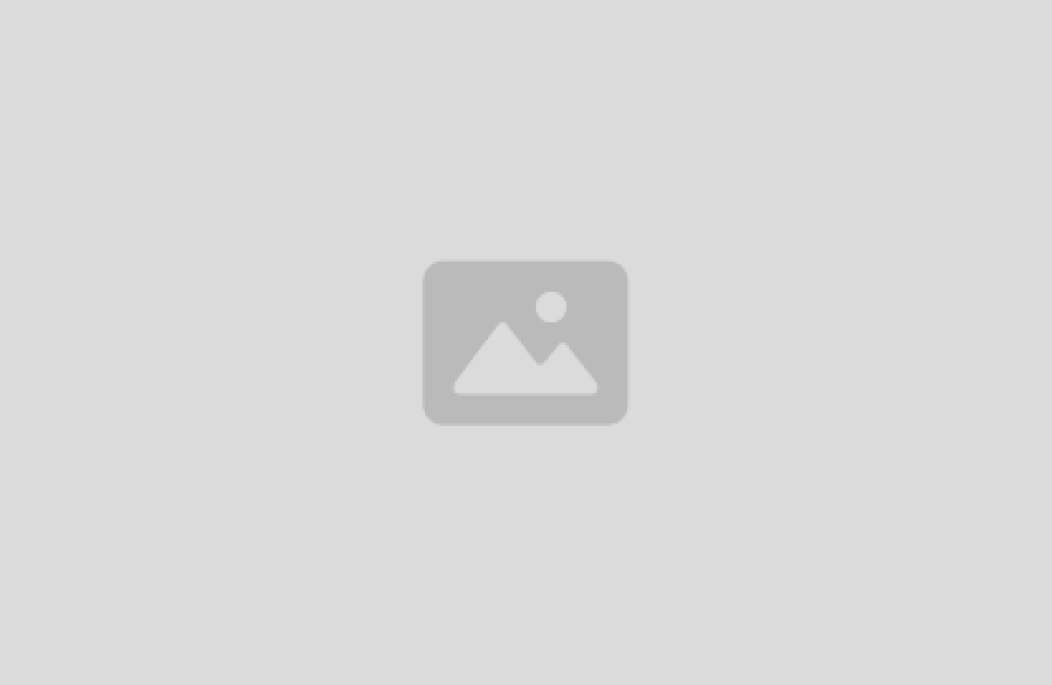
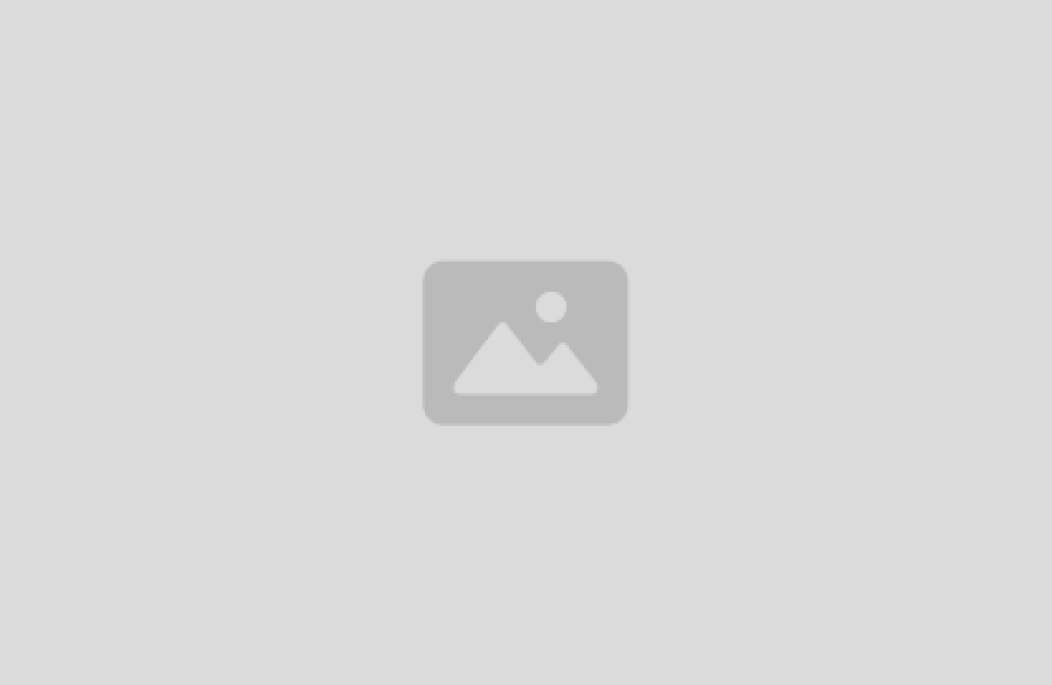
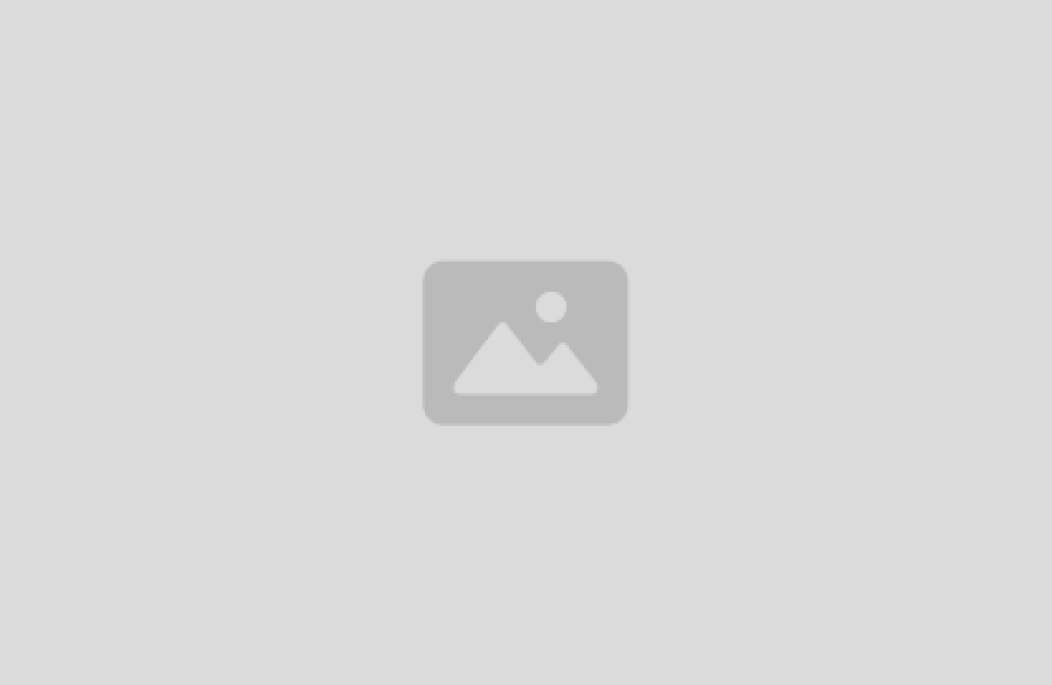
Discover more integrations
No items found.
Get in touch CTA Section
Lorem ipsum dolor sit amet, consectetur adipiscing elit, sed do eiusmod tempor incididunt ut labore et dolore magna aliqua.
Frequently asked questions
Can container-based environments improve incident response for data teams?
Absolutely. Containerized environments paired with observability tools like Kubernetes and Prometheus for data enable faster incident detection and response. Features like real-time alerts, dynamic thresholding, and on-call management workflows make it easier to maintain healthy pipelines and reduce downtime.
What role does technology play in supporting data team well-being?
The right technology can make a big difference. Adopting observability tools that offer features like data lineage tracking, data freshness checks, and pipeline health dashboards can reduce manual firefighting and help your team work more autonomously. This not only improves productivity but also makes day-to-day work more enjoyable.
Why is using WHERE instead of HAVING so important for performance?
Using WHERE instead of HAVING when not working with GROUP BY clauses is crucial because WHERE filters data earlier in the query execution. This reduces the amount of data processed, which improves query speed and supports better metrics collection in your observability platform.
What is “data-quality-as-code”?
Data-quality-as-code (DQaC) allows you to programmatically define and enforce data quality rules using code. This ensures consistency, scalability, and better integration with CI/CD pipelines. Read more here to find out how to leverage it within Sifflet
Why is data observability essential for building trusted data products?
Great question! Data observability is key because it helps ensure your data is reliable, transparent, and consistent. When you proactively monitor your data with an observability platform like Sifflet, you can catch issues early, maintain trust with your data consumers, and keep your data products running smoothly.
How does Sifflet support SLA compliance and proactive monitoring?
With real-time metrics and intelligent alerting, Sifflet helps ensure SLA compliance by detecting issues early and offering root cause analysis. Its proactive monitoring features, like dynamic thresholding and auto-remediation suggestions, keep your data pipelines healthy and responsive.
Can I monitor my BigQuery data with Sifflet?
Absolutely! Sifflet’s observability tools are fully compatible with Google BigQuery, so you can perform data quality monitoring, data lineage tracking, and anomaly detection right where your data lives.
How does data observability differ from traditional data quality monitoring?
Great question! While data quality monitoring focuses on detecting when data doesn't meet expected thresholds, data observability goes further. It continuously collects signals like metrics, metadata, and lineage to provide context and root cause analysis when issues arise. Essentially, observability helps you not only detect anomalies but also understand and fix them faster, making it a more proactive and scalable approach.