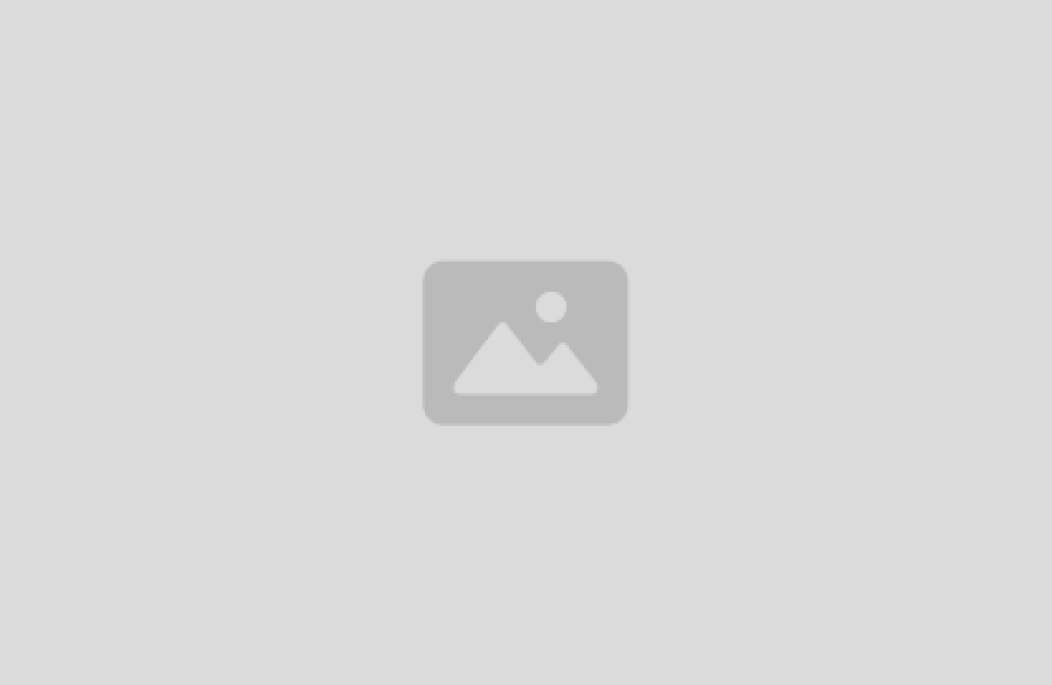
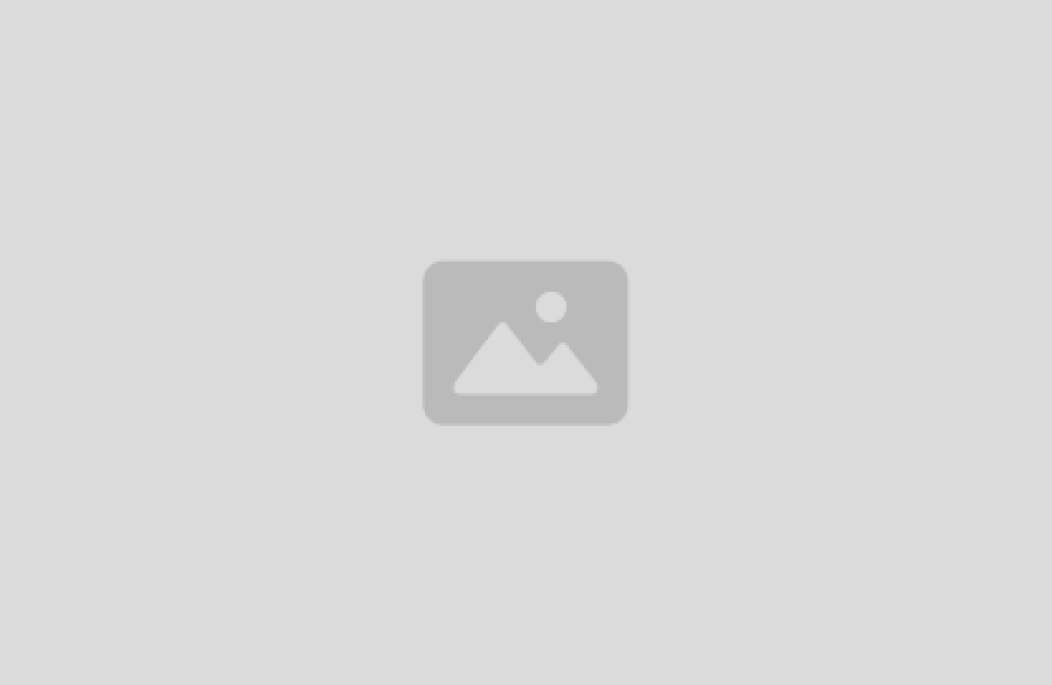
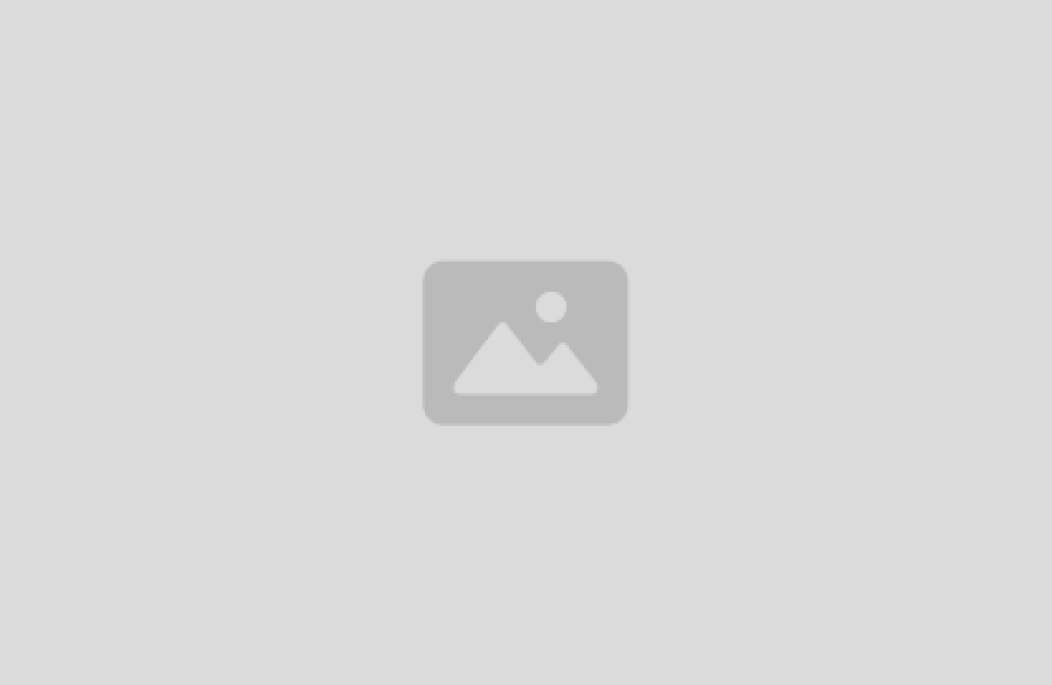
Discover more integrations
No items found.
Get in touch CTA Section
Lorem ipsum dolor sit amet, consectetur adipiscing elit, sed do eiusmod tempor incididunt ut labore et dolore magna aliqua.
Frequently asked questions
What’s new in Sifflet’s data quality monitoring capabilities?
We’ve rolled out several powerful updates to help you monitor data quality more effectively. One highlight is our new referential integrity monitor, which ensures logical consistency between tables, like verifying that every order has a valid customer ID. We’ve also enhanced our Data Quality as Code framework, making it easier to scale monitor creation with templates and for-loops.
Why is data observability important in a modern data stack?
Data observability is crucial because it ensures your data is reliable, trustworthy, and ready for decision-making. It sits at the top of the modern data stack and helps teams detect issues like data drift, schema changes, or freshness problems before they impact downstream analytics. A strong observability platform like Sifflet gives you peace of mind and helps maintain data quality across all layers.
How do I choose the right organizational structure for my data team?
It depends on your company's size, data maturity, and use cases. Some teams report to engineering or product, while others operate as independent entities reporting to the CEO or CFO. The key is to avoid silos and unclear ownership. A centralized or hybrid structure often works well to promote collaboration and maintain transparency in data pipelines.
What tools can help me monitor data consistency between old and new environments?
You can use data profiling and anomaly detection tools to compare datasets before and after migration. These features are often built into modern data observability platforms and help you validate that nothing critical was lost or changed during the move.
Can container-based environments improve incident response for data teams?
Absolutely. Containerized environments paired with observability tools like Kubernetes and Prometheus for data enable faster incident detection and response. Features like real-time alerts, dynamic thresholding, and on-call management workflows make it easier to maintain healthy pipelines and reduce downtime.
Can Sifflet extend the capabilities of dbt tests for better observability?
Absolutely! While dbt tests are a great starting point, Sifflet takes things further with advanced observability tools. By ingesting dbt tests into Sifflet, you can apply powerful features like dynamic thresholding, real-time alerts, and incident response automation. It’s a big step up in data reliability and SLA compliance.
How did implementing a data observability platform impact Hypebeast’s operations?
After adopting Sifflet’s observability platform, Hypebeast saw a 204% improvement in data quality, a 178% increase in data product delivery, and a 75% boost in ad hoc request speed. These gains translated into faster, more reliable insights and better collaboration across departments.
Why is data observability important when using ETL or ELT tools?
Data observability is crucial no matter which integration method you use. With ETL or ELT, you're moving and transforming data across multiple systems, which can introduce errors or delays. An observability platform like Sifflet helps you track data freshness, detect anomalies, and ensure SLA compliance across your pipelines. This means fewer surprises, faster root cause analysis, and more reliable data for your business teams.