Data Lineage: Moving from fire fighting to fire prevention.
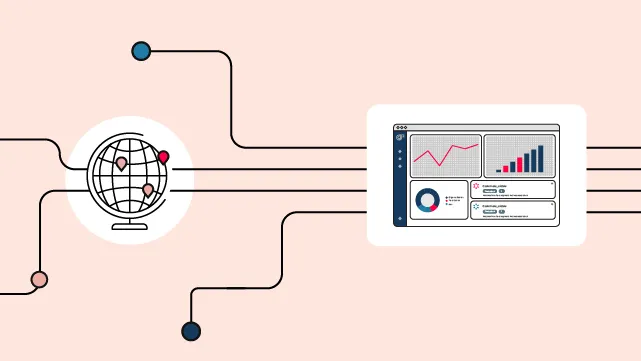
As more and more organizations adopt a data-driven culture and embrace processes and tools to scale the use of data within their teams, lineage is increasingly becoming an essential pillar of businesses’ data governance practices.
Lineage is the process of understanding and keeping track of data as it flows from data sources to consumption through the multiple transformations it goes through. This technology is now also the backbone of data observability tools. Easy, right? Not really. Lineage is one of the most complex concepts in data management, drawing a lot of confusion to it. In this blog, I aim to make a bit more clarity on the topic and provide some concrete use cases.
Let’s start from the beginning - How can we define data lineage?
People commonly think of lineage as the representation of the journey of their data - origin, journey, flows, and so on. And indeed, lineage is the series of steps from the data source to business dashboards or reports and the transformations and calculations throughout the journey. Lineage is also much more than this. Lineage takes into account all the dependencies that exist in data environments. These could be data points depending on each other, KPIs, interfaces, and so on. Connected together, these elements create a huge net of dependencies, causing the very high complexity we face when dealing with lineage.
This complexity is key for business. For organizations, the possibility to quickly navigate data environments allows them to act more quickly and therefore be more successful from a change of incident management perspective.
What are the use cases of lineage?
Lineage is a technology rather than a product and is the underlying enabler of many data products and services, such as catalogs. More generally, lineage is the underlying technology of the following products:
- Troubleshooting
- Impact assessment
- Discovery
- Regulations
Let’s go through each one of them in detail.
Troubleshooting
When data users spot anomalies in their dashboards and reports, they immediately want to know the root cause of the issue. Is it a technical problem in the pipelines, or is it a business issue that requires immediate attention? Lineage allows teams to check the status of all upstream dependencies, enabling them to see where the data broke and allowing them to troubleshoot it quickly.
Impact assessment
Teams need to know the impact of the changes they want to make on the pipelines. This is because dashboards and reports are powered by tables that are sitting in the organization’s data storage. If a data engineer makes a change, it could lead to breaking pipelines and processes. In turn, this can be detrimental to the data quality.
Lineage allows data users to identify all the assets that can be potentially impacted by any changes and ensure that they can minimize the negative impact. On top of this, if there is an impact, lineage allows the engineers to identify the affected data and notify the users.
Discovery
Teams need to be able to identify and understand their data sources. Knowing which data is powering the dashboards can give helpful information to users on how to use it and whether it is possible to trust it.
Regulations
Lineage is a powerful tool to improve data governance, access rights management, and consequently helps teams comply with regulations such as GDPR. Lineage allows teams to understand and track access rights.
Automated lineage features like PII propagation can improve data security.
Lineage applied to a data quality/ observability use case
As we have seen before, organizations rely on lineage for various use cases - troubleshooting, impact assessment, discovery, and regulations. And with the rise of data observability as a category, the application of lineage was also extended to DataOps.
At Sifflet, we believe that having an extensive line of data is paramount to the success of any data quality monitoring framework. Capturing dependencies and understanding how data is transformed, produced, and used across the entire ecosystem is key to ensuring reliability.
Observability is a concept that comes from software and, before that, from control theory. The idea of software observability - with what companies like Datadog and Newrelic have created over the past decade - was to help software engineers get a better sense of what’s going on inside their applications and monitor the health status of the different components of the applications. The same thing can now be applied to data.
Prevention is key
Not thinking about data health can be dangerous for organizations. Technology can enable data engineers to be “firefighters” and fix any situation quickly. But if organizations want to be more strategic with their data, they need to be able to prevent incidents from happening in the first place. Some organizations focus on monitoring, which is already a good first step. But it is not enough. Organizations need to start caring about their data health by implementing prevention strategies from day one.
What factors should organizations consider when implementing lineage for a data quality use case?
To make the implementation of lineage successful, the first step is to change the mindset within the organization. Teams need to move from fire fighting to fire prevention mode. In other words, organizations need to change the way they do things to have space for the prevention process.
Practically, this means that data engineers should have something available for them to run impact assessments before every change they implement. Similarly, data architects should have something they can use to decide about major architectural changes in the organization.
Seeing detailed and accurate maps of dependencies is the only way to do real prevention. And ideally, the lineage should be automated. Nobody wants to waste their time by manually going through all the different steps the data went through, so automation is key, together with accuracy and detail.

How lineage looks like in an observability tool
Automated field-level lineage
Most lineage tools provide a view at the table level. This is useful for tasks like finding the root cause of an issue and understanding dependencies.
Tools like Sifflet, also offer field-level view. Field-level lineage enables teams to understand the impact of changes as they relate to columns that are shared across tables, consequently narrowing down the scope of the investigation. On top of this, field-level lineage makes it easier to conduct root cause and impact analysis for data issues.
With this feature, data users can make table changes without worrying about losing trust in their data at each stage of the lifecycle.

Incident management and business impact assessment
Your lineage solutions should also have capabilities to allow you to assign, prioritize and categorize all alerts to optimize issues resolution. In this way, the team can assign owners and ensure that all involved stakeholders are in the know regarding the resolution of the issue.
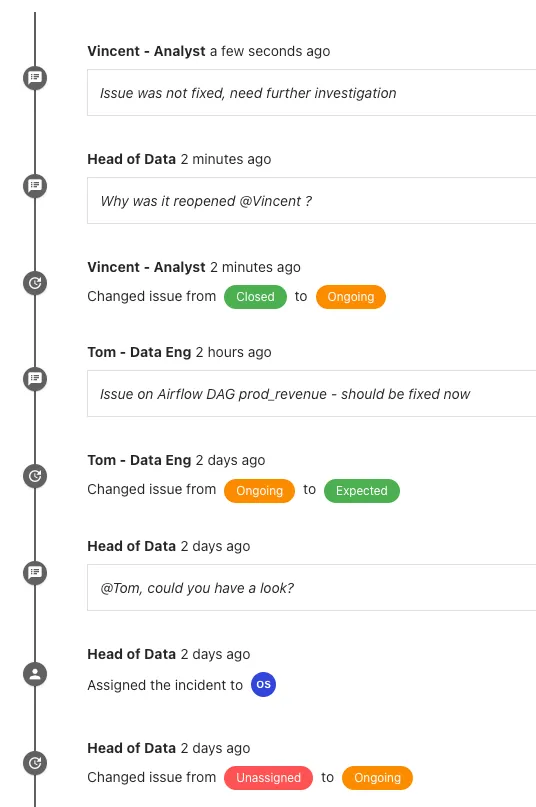
Root cause analysis
When the data breaks, it is essential that the team can assess the incident timeline and trace the incident upstream and downstream, across all of the tools of the modern data stack.
Conclusion - Obtaining a bird’s eye view of your data flow
Only a few years ago, lineage was about tracing relationships in the warehouse or lake. Now, lineage is cross-system, with tables powering BI tools like Looker, Tableau, and PowerBI. Today technical and business users can check where the data came from and ensure that no bad data is impacting the business. On top of this, end-to-end lineage - from ingestion to consumption - is now possible because most BI tools have APIs and perform SQL queries in a standardized way in the warehouse.
Lineage is one of the pillars of data observability, and without any doubt, goes hand in hand with data quality and discovery. Mapping out the dependencies between data enables users to troubleshoot any issue quickly and constantly maintain high reliability of their assets.